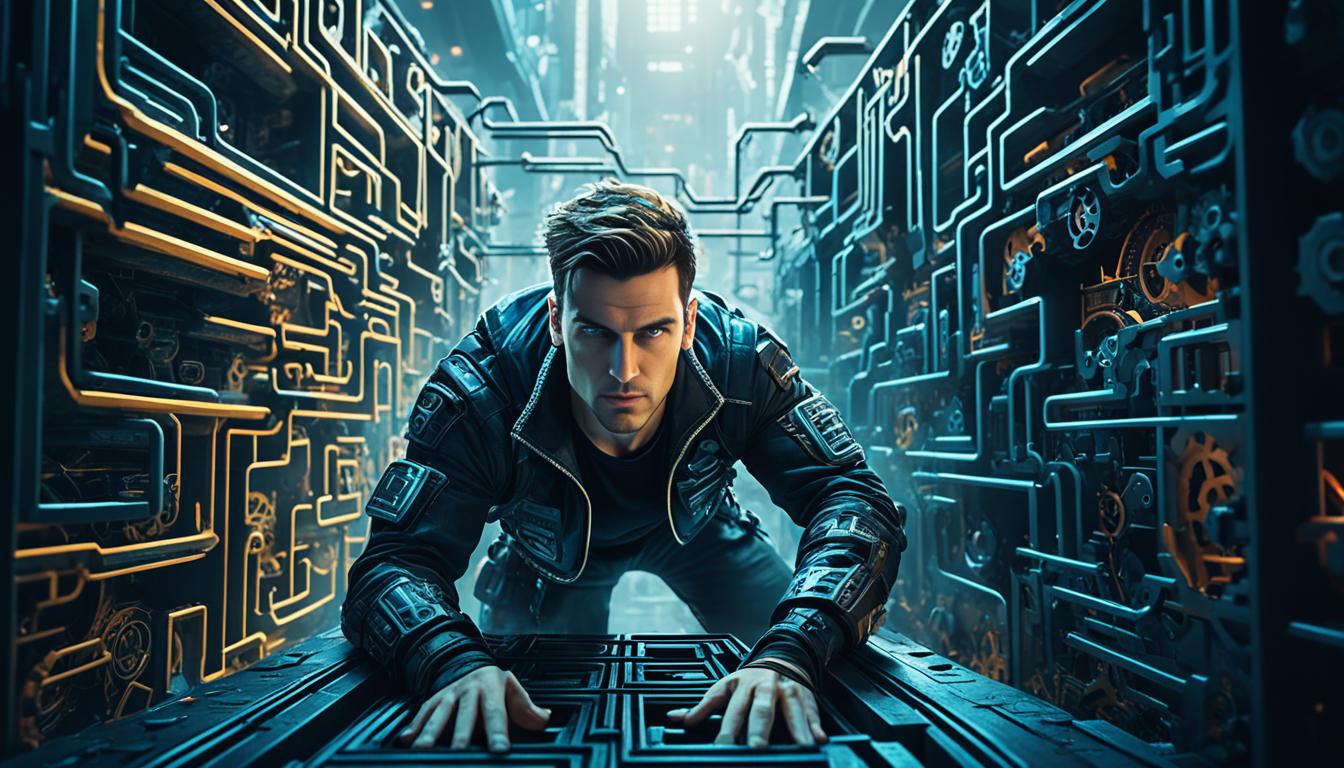
As the adoption of artificial intelligence (AI) continues to soar, with Forrester estimating that almost 100% of organizations will be using AI by 2025 and the AI software market reaching $37 billion, there is a growing concern about the potential for algorithmic bias. Algorithmic bias refers to situations where AI makes decisions that are systematically unfair to particular groups of people. Researchers have found that AI bias has the potential to cause real harm, as it can perpetuate and exacerbate existing societal inequalities.
The root of the problem lies in the fact that human beings choose the data that algorithms use and decide how the results of those algorithms will be applied. Without extensive testing and diverse teams, it is easy for unconscious biases to enter machine learning models, and then AI systems automate and perpetuate those biased models. Several studies have found examples of AI bias in real-world applications, such as facial recognition misidentifying people of color and mortgage algorithms charging higher interest rates for Latino and Black borrowers.
Key Takeaways
- AI adoption is expected to reach 100% by 2025, but concerns about algorithmic bias are growing.
- Algorithmic bias can perpetuate and exacerbate existing societal inequalities.
- Unconscious biases can easily enter machine learning models, leading to biased AI decisions.
- Real-world examples of AI bias include facial recognition and mortgage algorithms exhibiting racial bias.
- Addressing algorithmic bias requires a multifaceted approach involving developers, companies, and the broader society.
Understanding the Challenges of Algorithmic Bias in AI
As the adoption of artificial intelligence (AI) systems continues to accelerate, a growing concern has emerged around the issue of algorithmic bias. Algorithmic bias refers to situations where AI makes decisions that are systematically unfair to particular groups of people. This bias can arise from various sources, including the data used to train the algorithms, the algorithms themselves, and the way the models adapt and learn over time.
Defining Algorithmic Bias
Algorithmic bias occurs when the data used to train an AI model is not representative of the diverse populations the model will serve, leading to imbalances and biases in the model’s decision-making process. This data bias can then be amplified and perpetuated as the algorithm learns and makes decisions, resulting in discriminatory outcomes that reflect pre-existing societal inequalities.
Types of Algorithmic Bias
Algorithmic bias can manifest in various forms, including gender bias in recruitment algorithms, racial bias in facial recognition software, and disparities in the treatment of different socioeconomic groups. These biases can arise at different stages of the AI development process, from data collection and model training to deployment and adaptation in real-world environments.
Real-World Impacts of Algorithmic Bias
The deployment of biased AI models can have far-reaching, negative consequences, perpetuating stereotypes, infringing upon individual rights, and eroding public trust in technological advancements. Biased algorithms can affect job prospects, societal inclusion, and equal treatment under the law and in service delivery, leading to discriminatory outcomes that reinforce and exacerbate existing inequalities. High-profile cases, such as gender bias in recruitment algorithms and racial bias in facial recognition software, have highlighted the urgent need to address algorithmic biases and ensure the development of equitable and just AI systems.
How can I address concerns about algorithmic bias in AI systems by 2025?
Addressing biases in machine learning is a shared, ethical responsibility with significant implications. Developers are central in integrating fairness from conception to deployment; companies must foster ethical, transparent, and inclusive AI development environments, and end-users, along with the broader society, should advocate for equitable AI systems. Achieving fairness in machine learning and ethical AI development involves recognizing and addressing biases in every stage of the process, ensuring models produce equitable, unbiased, and inclusive results.
Inclusive Data Representation
This includes employing techniques to detect biases, such as using fairness indicators and model evaluation methods, and implementing mitigation strategies. Fairness indicators offer quantitative ways to measure model performance across diverse groups, identifying disparities in model predictions, while various model evaluation techniques, including confusion matrices and ROC curves, allow for comprehensive assessments of models’ fairness and biases. These techniques enable developers to uncover implicit, unintended biases, allowing for informed adjustments and refinements to address identified disparities, thereby contributing to the enhancement of model fairness and the reduction of harmful biases.
Algorithmic Auditing and Bias Testing
Detecting biases is a crucial step in building fair AI systems. Fairness indicators offer quantitative ways to measure model performance across diverse groups, identifying disparities in model predictions, while various model evaluation techniques, including confusion matrices and ROC curves, allow for comprehensive assessments of models’ fairness and biases. These techniques enable developers to uncover implicit, unintended biases, allowing for informed adjustments and refinements to address identified disparities, thereby contributing to the enhancement of model fairness and the reduction of harmful biases.
AI Governance Frameworks
The responsibility to address biases in AI systems also requires the development of robust AI governance frameworks. This includes drafting comprehensive AI use policies that outline ethical guidelines, data protection measures, and risk management strategies. Conducting regular privacy impact assessments (PIAs) to identify potential privacy risks associated with AI projects is also critical. Ensuring transparency and obtaining meaningful user consent are essential, as is implementing robust data security measures to protect personal information used in AI systems. Staying updated on evolving regulations and standards, and fostering a culture of privacy and responsible AI practices within the organization, are also key strategies to address concerns about algorithmic bias in AI systems by 2025.
Conclusion
As AI continues to advance and become more integrated into various aspects of our lives, the need to address concerns about algorithmic bias becomes increasingly urgent. Developing fair and ethical AI systems requires a multifaceted approach involving developers, companies, and the broader society. By ensuring inclusive data representation, implementing rigorous algorithmic auditing and bias testing, and establishing comprehensive AI governance frameworks, we can work towards mitigating the risks of algorithmic bias and unlocking the full potential of AI to create a more equitable and just future.
Continuous vigilance, multi-stakeholder collaboration, and a steadfast commitment to responsible AI principles are essential to address concerns about algorithmic bias in AI systems by 2025 and beyond. Only through a collective effort can we ensure that the transformative power of AI is harnessed in a way that promotes fairness, inclusion, and justice for all.
As we navigate the rapidly evolving landscape of AI, let us remain vigilant, empowered, and united in our pursuit of a future where technology serves as a catalyst for positive change, empowering individuals and communities, and fostering a more equitable and just world.
FAQ
What is algorithmic bias and why is it a concern?
Algorithmic bias refers to situations where AI makes decisions that are systematically unfair to particular groups of people. It can occur due to pre-existing biases in the data used to train the algorithms, limitations or characteristics of the algorithms themselves, or the way the models adapt and learn over time. Algorithmic bias can have far-reaching, negative consequences, perpetuating stereotypes, infringing upon individual rights, and engendering mistrust in technological advancements.
How can algorithmic bias infiltrate machine learning systems?
Bias can infiltrate ML systems at various developmental stages, including data collection, model training, and model deployment. During data collection, bias can be introduced if the collected data is not representative of the diverse populations the model will serve or if it contains inherent biases reflecting pre-existing inequalities in society. At the model training stage, bias can be introduced through the algorithm’s design or through biased training data, leading the model to learn and perpetuate discriminatory patterns. Even if a model is trained with unbiased data and algorithms, its interaction with real-world data and dynamic environments during deployment can lead to the emergence of new biases or the reinforcement of existing ones, affecting the model’s fairness and accuracy in unforeseen ways.
What are some examples of real-world algorithmic bias?
Several studies have found examples of AI bias in real-world applications, such as facial recognition misidentifying people of color and mortgage algorithms charging higher interest rates for Latino and Black borrowers. These instances of gender bias in recruitment algorithms and racial bias in facial recognition software have highlighted the need to address algorithmic biases to ensure the development of equitable and just AI systems.
How can I address concerns about algorithmic bias in AI systems by 2025?
Addressing biases in ML is a shared, ethical responsibility with significant implications. Developers are central in integrating fairness from conception to deployment; companies must foster ethical, transparent, and inclusive AI development environments, and end-users, along with the broader society, should advocate for equitable AI systems. This includes employing techniques to detect biases, such as using fairness indicators and model evaluation methods, and implementing mitigation strategies like ensuring inclusive data representation, conducting algorithmic auditing and bias testing, and establishing comprehensive AI governance frameworks.
What are the key strategies for mitigating algorithmic bias in AI systems?
Key strategies for mitigating algorithmic bias in AI systems by 2025 include:
– Ensuring inclusive data representation to address data biases
– Implementing rigorous algorithmic auditing and bias testing techniques
– Developing robust AI governance frameworks with ethical guidelines, data protection measures, and risk management strategies
– Fostering a culture of privacy and responsible AI practices within organizations
– Ensuring transparency and obtaining meaningful user consent
– Staying updated on evolving regulations and standards
– Promoting multi-stakeholder collaboration to address algorithmic bias concerns